
Artificial Intelligence
We build the first AI-powered battery intelligence
Fast-Track Battery Innovation through AI-Generated Synthetic Data
Traditional battery modelling is slow and resource-intensive. Our AI-driven approach reduces time and cost by generating synthetic data for all key performance and lifetime modalities. This enables rapid prototyping, faster iteration cycles, and improved decision-making for R&D and production teams.
Try out Sphere’s APEX Model
We’ve developed the first generative pre-trained transformer model for battery development using over 4,000 battery cell tests to predict end-of-life aging and performance. Unlock faster development by leveraging your data with a company-wide battery AI model.
Run a Proof of Concept with us
We offer a structured approach to testing our technology with your battery data:
Pre-Trained Model Deployment: Leverage our existing AI-driven Product EXcellence models for quick initial evaluations.
POC Collaboration: Work with us to test the simulation power on your data.
Model Deployment & Customization: Fine-tune the AI model based on your specific battery systems.
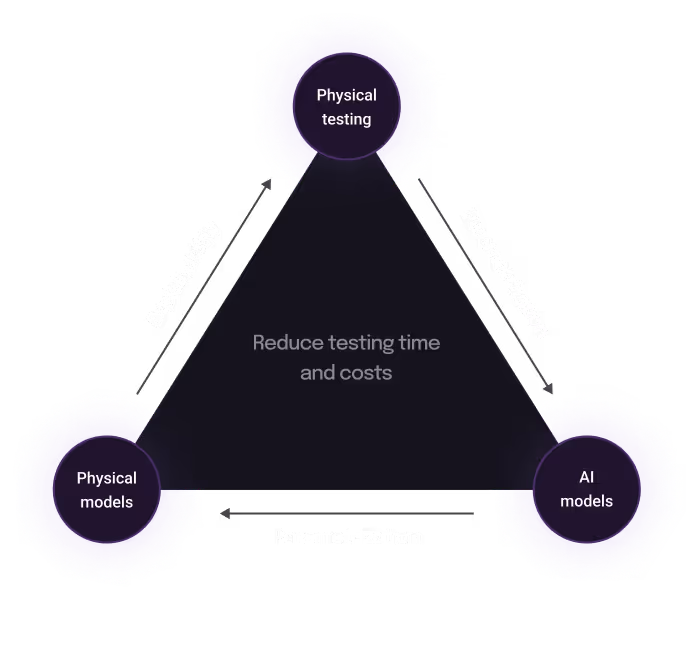
Key Benefits for Your Organization
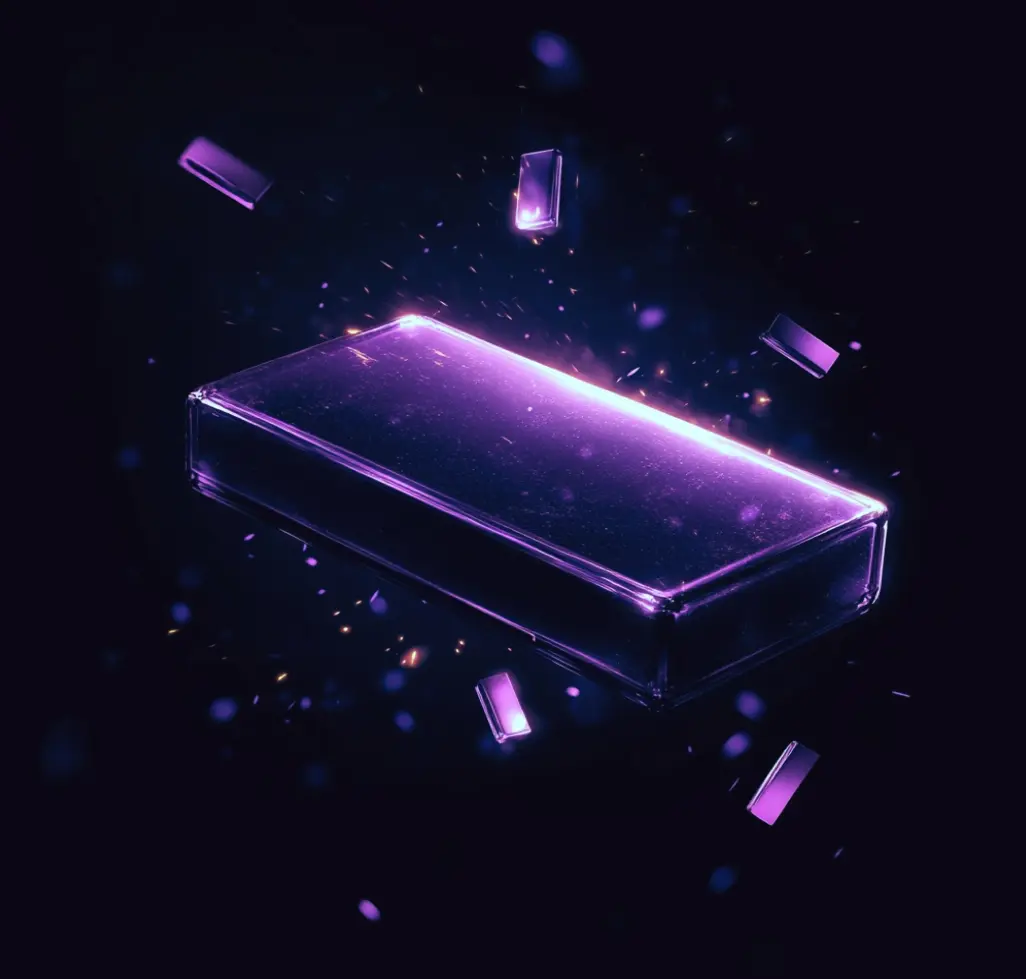
Reduce Time & Cost
Minimize expensive physical testing through AI-generated synthetic data.
Comprehensive Performance Insights
Predict battery behaviour across multiple conditions, including aging, temperature, and performance shifts.
Scalability
Applicable across different cell chemistries with minimal adjustments.
Seamless, Scalable, Smart: Managing
Battery Data with AI
We provide end-to-end solutions to help you establish and maintain a seamless data ecosystem.
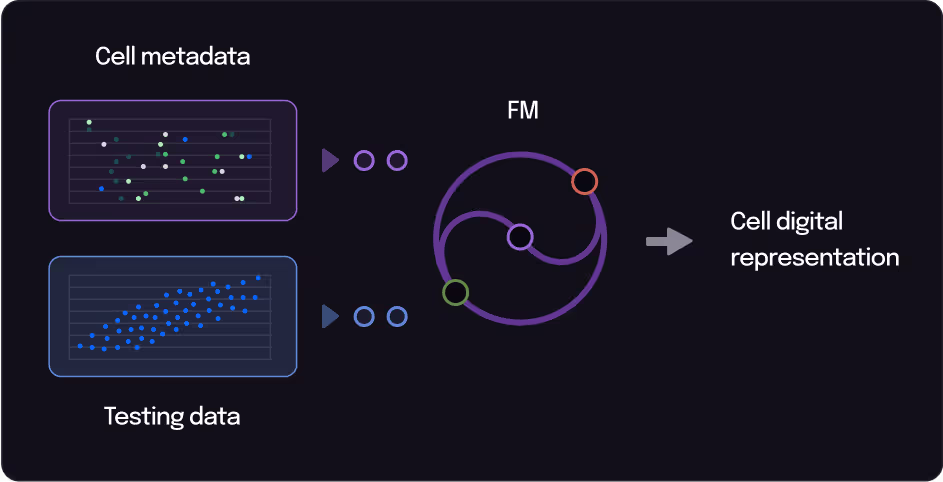
Time-Series Foundation Models
Our AI combines both static (cell properties, material specs) and dynamic (cycling data, environmental conditions) data to build a robust foundation model.
From Prediction to Perfection - AI for Battery Development
Our approach draws inspiration from Large Language Models (LLMs) and adapts their architecture to process time-series data efficiently. Just as LLMs predict word sequences, our AI predicts battery performance and degradation over time.

1. Smart testing strategy
Innovative high-accuracy tests informed by AI model.
2. AI simulation output
High-fidelity battery simulations powered by AI models.
4. Bayesian optimization: next best test
AI-guided to identify the next best test.
3. Confidentiality analysis of simulation
Secure analysis ensuring simulation data confidentiality.
Results of Sphere's APEX Simulation Model
- Simulate new cell type
Predicting the discharge capacity fade over time
Predicting voltage fade at any given cycle
- Simulate new current profile
Smarter, Faster, More Scalable
Our method surpasses both traditional ECM/FEM simulations and pure deep learning models by offering:
Pre-Trained Model Deployment: Leverage our existing AI models for quick initial evaluations.
POC Collaboration: Work with us to test the simulation power on your data.
Model Deployment & Customization: Fine-tune the AI model based on your specific battery systems.
Traditional Modeling Modelling of specific battery cells based on ECM or Finite-Elemente-Methode | Sphere Foundation AI Approach Foundation AI based multi chemistry modelling.. | |
---|---|---|
Model Training Complexity | High Requires detailed understanding of physics and chemistry, complex meshing, and material properties. | Moderate to High Requires large datasets for training, but less domain-specific expertise once the AI model is pre-trained. |
Input Data Needed to Fine-Tune Models | Physical & chemical data Detailed experimental data, material properties and cell design specs | Large-scale time series data Historical usage data, charge/discharge cycles, environmental conditions. |
Simulation Speed | Slow FEM sim are computationally intensive, especially for 3D models. ECMs are faster but less detailed. | Fast Once trained, AI models can simulate quickly, often in real-time or near real-time. |
Prediction Accuracy - General | High for specific phenomena Accurate when parameters are well-defined and calibrated. | Potentially higher and adaptive Improves with time & more data, capturing complex patterns and anomalies. |
Prediction Accuracy - State of Health (SOH) | High when specific degradation mechanisms are well-understood and modeled. | Potentially higher for long-term predictions, leveraging large datasets and continuous learning. |
Self learning capabilities | None every model needs to be trained again when design changes are introduced | High the model grows over time and implements learning to improve its ability to predict performance and identify anomalies |
Include other (than time series data) sources into simulation | Not possible | High Including e.g.: tear down images, SEM of macroscopic features |
Generalizability Across Cell Chemistries | Limited requires re-parameterization and validation for different chemistries | High can generalize, applicable to various chemistries with minimal re-training. |
Supercharge Your Battery Innovation with AI